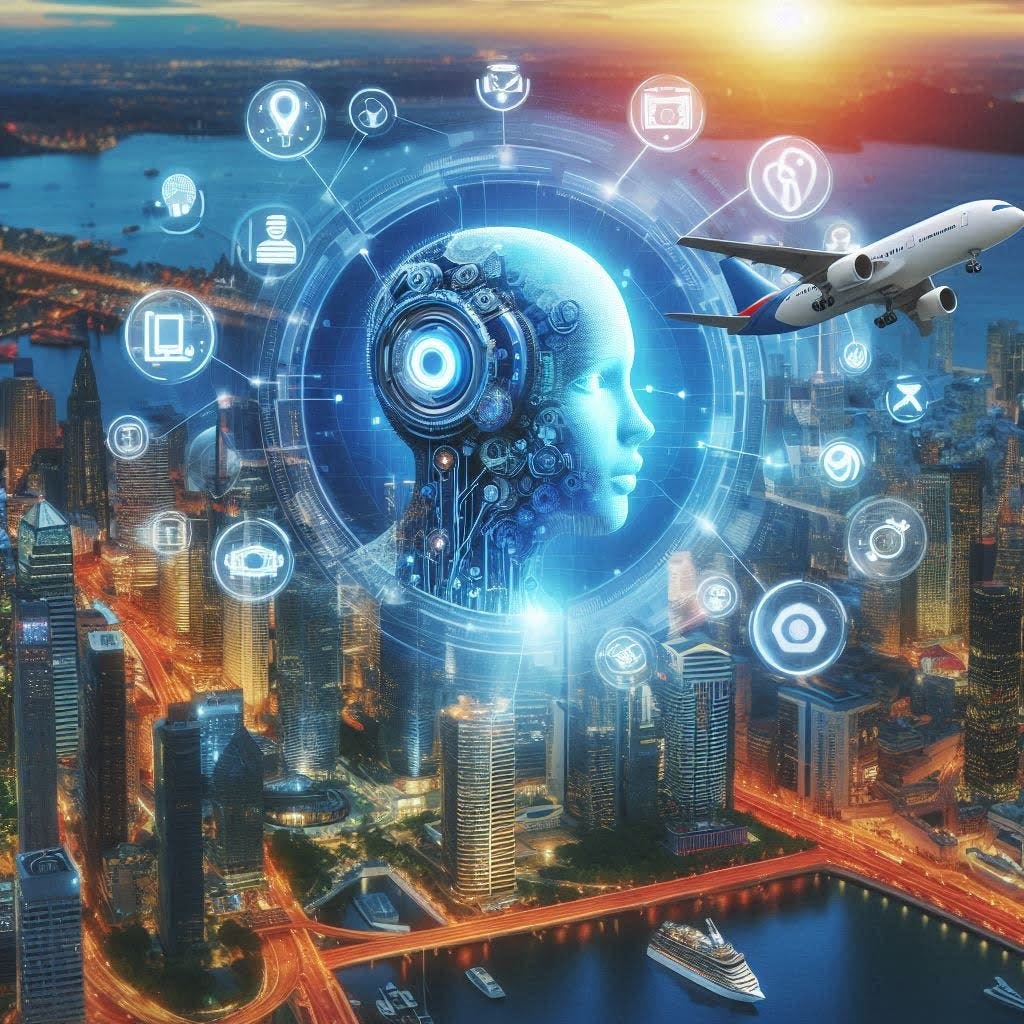
In an age defined by rapid technological advancement, we find ourselves at the intersection of two revolutionary forces: blockchain and artificial intelligence (AI). These technologies are converging to create decentralized agentic AI systems that promise to redefine how we engage with technology. With the potential to deliver unmatched autonomy, security, and efficiency, these solutions are not just innovations; they are the future of connectivity.
Understanding Decentralization
At the heart of these systems lies the principle of decentralization. Traditional models often rely on centralized authorities, but decentralized agentic AI operates on distributed networks, fundamentally transforming connectivity. This shift offers a multitude of advantages:
- Enhanced Security: Decentralization enhances security, making it significantly harder for malicious actors to infiltrate the entire network. Data is safeguarded across multiple locations, providing a robust defense against cyber threats.
- Data Integrity: In an era where data breaches are alarmingly common, blockchain’s design creates a secure environment for sensitive information. Every transaction is encrypted and recorded in an immutable ledger, protecting data integrity and preventing unauthorized access.
- Empowered Users: Users gain enhanced control over their data and interactions. By deploying intelligent agents in their environments, they reduce reliance on third-party services, fostering trust and ownership.
- Resilience Against Failures: By distributing workloads across numerous nodes, these networks eliminate single points of failure, ensuring uninterrupted service even if one node is compromised.
The Rise of Self-Sufficient Agents
Imagine a future where technology operates autonomously, learning and adapting to your preferences without constant oversight. This vision is becoming a reality with the advent of decentralized agentic AI systems:
- Autonomous Decision-Making: These systems learn from experiences and adapt to new situations, enhancing their effectiveness over time without the need for human intervention.
- Resource Management: Users take charge of their agents’ operations, similar to how car owners maintain their vehicles, encouraging a proactive approach to technology.
- Smart Contracts: By leveraging smart contracts, these agents can automate processes and enforce agreements without intermediaries, streamlining operations and boosting overall efficiency.
Embedded Blockchain AI Agents: A New Frontier
One of the most exciting developments in this landscape is the integration of embedded blockchain AI agents within devices. This technology allows everyday objects to operate autonomously and securely, bringing the power of decentralized intelligence to our homes and workplaces. Here’s how embedded agents are changing the game:
- Smart Devices: From smart thermostats to security cameras, embedded blockchain AI agents can analyze data in real time, making decisions based on user preferences and environmental conditions without needing a constant internet connection. This capability enhances efficiency and user experience while maintaining security.
- Improved Data Privacy: With data being processed locally on the device, sensitive information never has to leave the user’s environment, significantly reducing the risk of data breaches and enhancing privacy.
- Seamless Interoperability: Embedded agents can communicate with other devices on the network, creating a cohesive ecosystem that enhances functionality. This interoperability allows for smarter homes and offices where devices work together to optimize energy use, improve security, and streamline daily tasks.
Building Transparency and Trust
Transparency is essential in establishing trust, and blockchain technology inherently provides this advantage:
- Immutable Records: Every action taken by these agents is permanently recorded on the blockchain, allowing users to verify operations and ensuring accountability at every level.
- Proof of Performance: Mechanisms like Proof-of-Coverage validate that agents fulfill their commitments, allowing users to confirm that their systems deliver as promised.
- Decentralized Trust: This model fosters trust through collective validation of actions by the network rather than relying on a central authority, making it particularly appealing in industries where verification is essential.
Connecting Digital and Physical Realms
Decentralized agentic AI systems are crucial in bridging the gap between the digital and physical worlds through the Internet of Things (IoT) and other technologies:
- IoT Integration: These agents can interact seamlessly with various IoT devices, enabling real-time data exchange and collaborative decision-making, creating smarter environments from homes to industrial operations.
- Cross-Platform Collaboration: The systems can collaborate across different networks, enhancing operational capabilities and facilitating data exchange across both digital and physical domains.
- Standardized Protocols: Protocols like WHIP in the Helium network ensure efficient communication between devices and the blockchain, allowing agents to transmit data and receive instructions effortlessly.
Economic Models Driving Engagement
The operational dynamics of decentralized agentic AI introduce innovative economic models that encourage participation:
- Marketplace Dynamics: A competitive marketplace allows users to lease or purchase agentic AI solutions, driving down costs and improving service quality.
- Dynamic Pricing Models: Users can set prices for services, optimizing resource allocation based on demand and availability.
- Token Incentives: Native tokens reward users for their engagement in the network, promoting active involvement and contributions.
Embracing the Future of Agentic AI
As we delve into the operational dynamics of these decentralized systems, it’s clear we are on the verge of a significant transformation. The integration of blockchain and AI sets the stage for agents that not only operate independently but also collaborate seamlessly with other systems and users, effectively bridging the digital and physical worlds.
Imagine a future where these agents work together to solve complex problems, share resources, and enhance decision-making across various sectors. By cultivating a decentralized, transparent, and autonomous ecosystem, decentralized agentic AI is poised to redefine our relationship with technology.
Conclusion
The journey toward a decentralized future powered by agentic AI is already underway with blockchain technology leading the way. By embracing the operational dynamics of these advanced systems, companies like Synergetics.ai are unlocking new possibilities for innovation, efficiency, and collaboration. As we stand at the forefront of this new era, leveraging the power of decentralized agentic AI will reshape connectivity for generations to come. This transformation will not only enhance user experiences but also ensure the security and integrity of the data that drives our increasingly interconnected world.