The Missing Piece in Agentic AI
Everyone is talking about agentic AI systems — how they will revolutionize business, streamline automation, and enhance human-machine collaboration.
But almost no one is talking about the foundational challenge that will determine whether these systems succeed or fail: identity.
Right now, the AI agents being built by tech giants and startups alike are nameless, faceless, and transient. They exist for a moment—running a task, executing a script — before vanishing into the digital ether. This lack of identity means there is:
🚫 No traceability – No way to verify which AI agent performed an action.
🚫 No accountability – No mechanism to hold AI systems responsible for their decisions.
🚫 No trust – No persistent identity for agents to securely interact with humans or other AI systems.
And yet, trust is the bedrock of every system humans rely on — whether in financial transactions, business negotiations, or even basic communications. Without persistent, verifiable identity, AI systems will remain untrusted and unscalable.
We’ve tackled this problem head-on by creating AI agents that can be permanently identified, tokenized, and securely stored in a digital wallet.
Let’s dive into why this is the missing key in agentic AI — and why telcos, enterprises, and policymakers need to pay attention.
The Human Parallel: How Identity Works in the Real World
A human identity follows a clear, traceable lifecycle:
1️⃣ Birth – You are assigned a birth certificate that permanently registers your identity.
2️⃣ Life – You carry IDs (such as a driver’s license, passport, employee badge) to prove who you are in different contexts.
3️⃣ Transactions – You sign contracts, pay bills, and interact with others using your verified identity.
4️⃣ Death – A death certificate marks the end of your legal presence.
Now, compare this to today’s AI agents:
❌ No birth record – An AI agent is spun up at will, with no permanent ID.
❌ No verifiable transactions – There’s no universal way to prove which agent did what.
❌ No traceability – If an AI-generated deepfake spreads disinformation, there’s no way to track it back to its source.
This lack of continuity is the Achilles’ heel of AI systems. The solution? Tokenized, persistent identity.
How Tokenized Identity Solves the Trust Problem
In computer science, a daemon is a background process that runs continuously, often providing essential system functions without direct user interaction. Humans, in many ways, resemble long-running daemons — once born, we persist continuously until death, with an uninterrupted existence and a traceable identity from birth to death. Our identity is recorded, updated, and verified across systems, ensuring we are accountable for our actions throughout our lifetimes. However, AI agents do not function this way. Unlike humans, AI agents are not persistent by default — they can be spun up, perform a task, and shut down in seconds, leaving no inherent trace of their existence. A single AI agent might execute a financial transaction, generate a piece of content, or initiate a system action before disappearing, with no way to verify who — or what — was responsible for that action. Without permanent identity and traceability, AI agents exist as ephemeral, unaccountable entities, making them vulnerable to misuse, fraud, and manipulation.
This is precisely why tokenized AI identity is critical. If an AI agent executes a harmful action — whether due to a coding flaw, a bad actor’s manipulation, or unintended consequences — how do we track the responsible party? Without a persistent identifier, it becomes impossible to assign accountability, regulate AI behaviors, or create reliable auditing mechanisms. If a bot spreads misinformation, completes a fraudulent transaction, or executes an unauthorized system change, and then disappears upon shutdown, there is no trail leading back to its source. Tokenization solves this by ensuring that AI agents have a permanent, immutable identity — one that persists whether the agent is running or not. With tokenized AI, every action is traceable, every agent is accountable, and organizations can ensure responsible AI deployment. The Synergetics AgentWorks platform has implemented this at scale, ensuring that each AI agent, once created, has a lifelong, verifiable identity—a necessary step in making agentic AI systems secure, transparent, and fit for enterprise and global adoption.
At Synergetics.ai, we’ve developed a tokenization framework that permanently assigns a verifiable, blockchain-backed identity to every AI agent. We did the research, built what is at the moment the only one of its kind, and wouldn’t be as adamant about championing this product-centric approach if we didn’t see the tremendous societal value in:
📌 Tokenized Agents: Each AI agent is issued a unique, permanent ID upon creation.
📌 Blockchain Verification: The ID is stored on a secure ledger for full traceability.
📌 Zero-Knowledge Proofs (ZKP): Identity can be verified without exposing sensitive data—powered by Privado.ai’s ID framework.
📌 Wallet Storage: AI agents carry their identity in a digital wallet, just like humans carry passports and driver’s licenses.
This approach enables three critical functions for agentic AI:
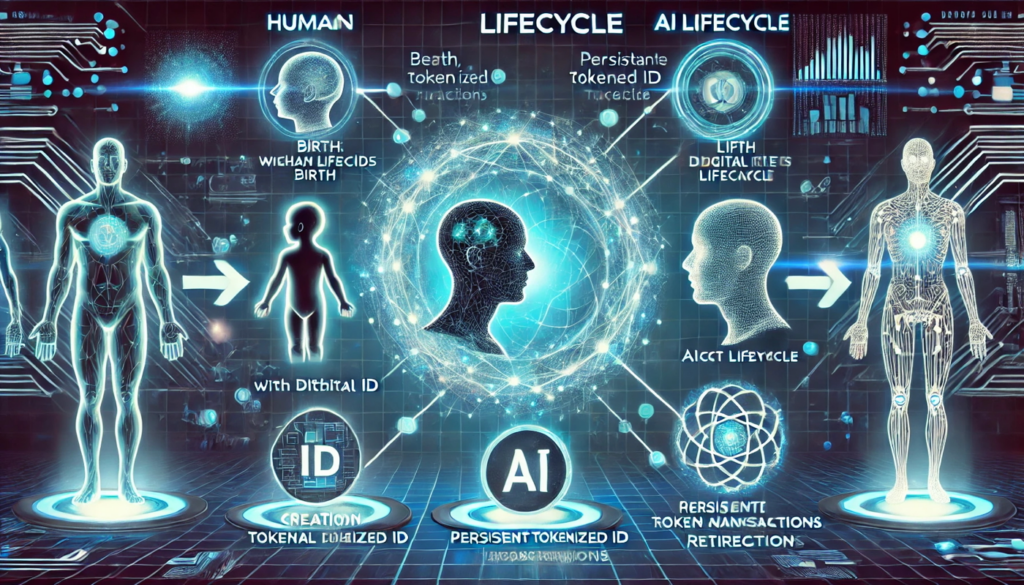
✅ Trust & Accountability – Enterprises can verify which AI agent made a decision or completed a transaction.
✅ Cross-Enterprise Communication – Agents can authenticate themselves when working across organizations.
✅ Security & Compliance – AI systems can meet regulatory and ethical requirements in enterprise and government applications.
The Role of AI Wallets: Storing and Managing Identity
If AI agents are to operate autonomously, they need more than just an identity — they need a secure way to store and use it.
This is where Agent Wallets come in.
🛠 AgentWallet is a secure digital storage for AI agent identity, assets, and credentials. Just as a human carries IDs and credit cards in a physical wallet, an AI agent must have a trusted place to store its identity and interact with the digital world.
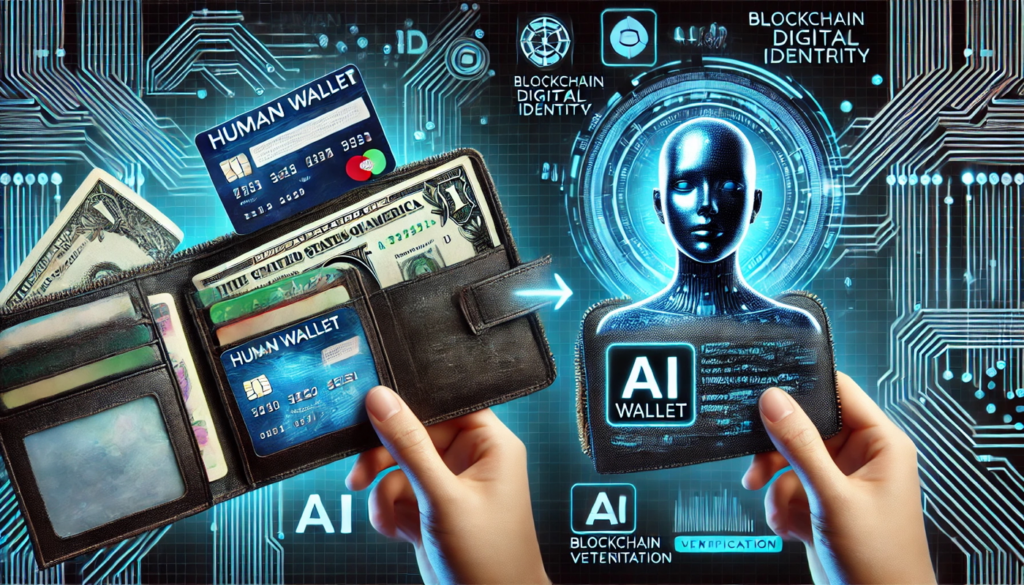
🔹 Key Features of an AI Wallet:
• Stores permanent agent identity
• Holds digital assets, cryptographic signatures, and credentials
• Allows for seamless authentication across enterprises
• Enables secure transactions between AI agents
Enterprise vs. Public Identity: A Two-Tiered System
Just as humans carry different forms of ID, AI agents will require two distinct identity types: an enterprise ID and a public ID.
In the same way that a person receives a state-issued ID — such as a driver’s license — to verify their identity within their home state or country, an AI agent operating within an enterprise must also have a verifiable enterprise ID to authenticate itself in internal systems. This enterprise ID ensures that the AI agent is recognized, trusted, and authorized to perform specific functions within the organization’s secure, private network. However, when a human crosses international borders, their state-issued ID is no longer sufficient — they need a passport to validate their identity across countries. Similarly, when an AI agent needs to operate outside its enterprise, interacting with external AI agents, digital services, or other organizations, it requires a public ID.
This public, blockchain-backed identity serves as a decentralized verification mechanism, ensuring that the agent is authenticated and trusted beyond its original enterprise environment. Just as a passport provides proof of identity, nationality, and authorization for international travel, an AI agent’s public ID enables it to securely interact with external systems, negotiate transactions, and build verifiable trust in agent-to-agent communications.
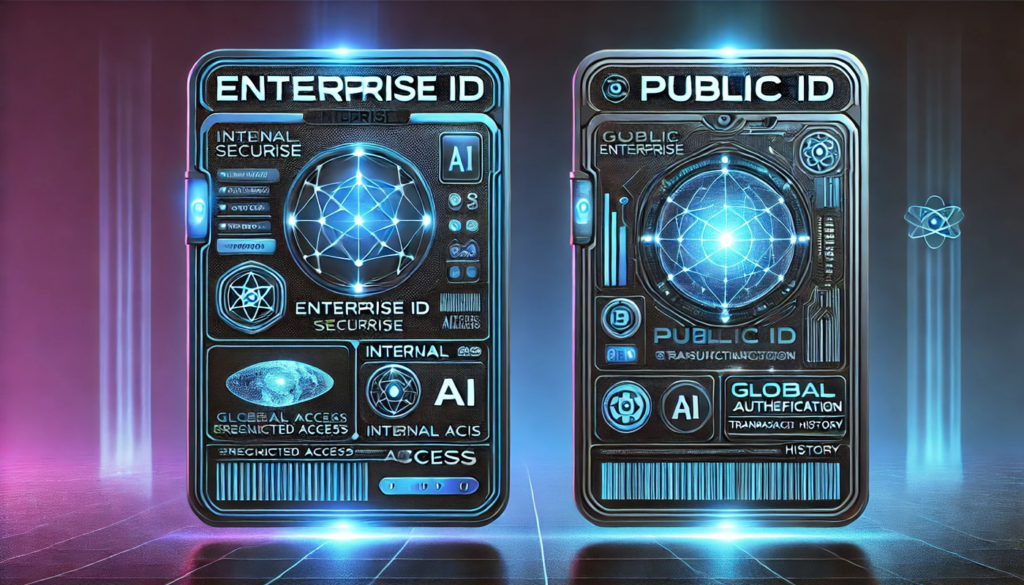
1️⃣ Enterprise ID (Private Blockchain)
🔹 Issued within a company for internal AI agents
🔹 Ensures secure transactions & compliance
🔹 Operates on Hyperledger Fabric or similar private blockchains
2️⃣ Public ID (Decentralized Ledger)
🔹 Allows AI agents to interact outside the enterprise
🔹 Used for cross-company AI negotiations, digital commerce
🔹 Runs on a public blockchain for transparency & verification
Without this dual-identity model, AI agents will be restricted in scope — unable to operate securely outside their original environment.
Why Telcos & Enterprises Must Act Now
The identity problem in AI isn’t a theoretical issue — it’s already playing out in real-world security concerns:
🚨 AI Deepfakes – Bots impersonate real people, spreading misinformation.
🚨 Automated Fraud – AI agents execute unauthorized financial transactions.
🚨 Data Leaks & Privacy Risks – Anonymous AI agents collect and misuse user data.
By adopting tokenized identity and AI wallets, enterprises and telcos can:
✔ Ensure traceability in AI-driven decisions
✔ Secure agent-to-agent communications
✔ Meet evolving AI governance & compliance standards
Final Thought: AI Identity is a Make-or-Break Issue
AI systems are evolving fast, but trust will determine their adoption. The next step? Embedding identity into the DNA of agentic AI. This will provide individual and enterprise users with:
✅ Permanent, blockchain-backed identity
✅ Secure, verifiable agent transactions
✅ Wallets for AI to store credentials & assets