Remember when AI was just about Roombas cleaning your floors or using voice commands on your computer? Today, AI has come so much further, especially in healthcare! It’s helping doctors, nurses, and clinics run smoothly while giving patients better care. Healthcare autonomous agents are programs designed to do specific work, learn from data, and automate processes.
Synergetics’ healthcare-focused AI agents are part of their Agentic AI platform, which allows healthcare providers to create and implement specialized AI solutions effortlessly. The Synergetics platform offers LangCertify, which ensures regulatory compliance, and AgentFlow, which enables real-time data integration and insights. These technologies help improve patient satisfaction and efficient operations for doctors and nurses.
In this blog, we will learn what AI agents manage and why they’re so useful. Keep reading and learn more!
What are AI agents in Healthcare?
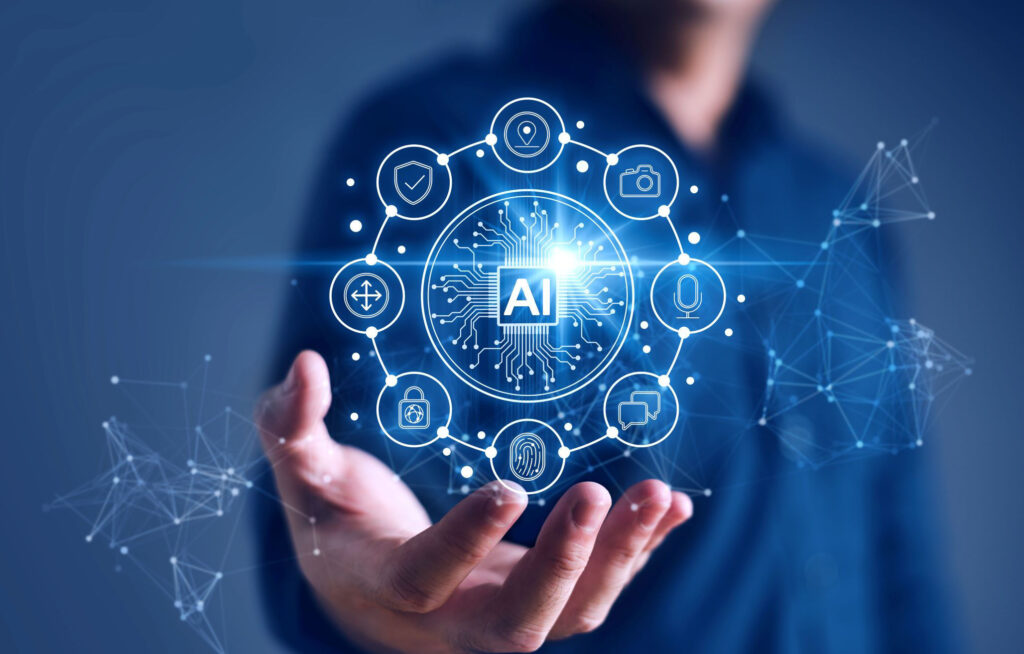
Artificial intelligence in healthcare is a blessing. These agents are like digital assistants in the medical sector, able to perform various tasks, from managing patient data to analyzing medical information. These agents improve management processes and help medical professionals make decisions. Certain AI agents, for instance, can track a patient’s health, while others may help radiologists by analyzing medical photos.
Moreover, AI agents can monitor patients’ health and identify potential risks early, alerting healthcare providers when medical attention is required. AI chatbots are always ready to provide medical help whenever needed. This increases patient satisfaction while simultaneously decreasing the workload for doctors and nurses.
As AI technology develops, we will see even more fascinating developments in healthcare. In fact, a survey found that 79% of healthcare professionals believe that robotics and AI will significantly improve the healthcare industry.
Synergetics products allow healthcare providers to create and deploy AI agents quickly, contributing to the seamless introduction of these agents into current medical systems.
Key benefits of AI agents in healthcare
If you’re considering implementing AI in the medical sector, understanding the advantages is essential. Here are some key benefits that tell how AI is vital for the medical sector:
- Data Management
AI automation agents can handle large amounts of data in just a few seconds, sorting through patient records and medical histories. Hence, doctors or nurses have more time to focus on the patient. Improved data management also means more accurate patient records and better compliance with regulations, making healthcare providers more efficient overall.
- Virtual Nursing Assistants
AI virtual nurse assistants, including chatbots and mobile apps, are great tools for answering questions about medications, sending reports to doctors, and helping with appointment scheduling. A recent study found that 64% of patients feel comfortable using AI for ongoing support, which can enhance nursing services.
By managing routine tasks, virtual nursing assistants ease the burden on clinical staff. These technological changes show how technology can support healthcare workers while improving patient satisfaction.
- Protection from Fraudulent
Adding AI to fraud prevention can greatly help insurance companies detect unusual or suspicious patterns in claims. AI is capable of identifying red flags, such as charging for expensive services that were never provided, examining bills for services that need to be bundled, or asking pointless tests to maximize insurance payouts. Artificial intelligence (AI) systems can quickly and efficiently analyze vast volumes of data using advanced algorithms and machine learning. This makes it simpler to spot possible fraud before it becomes a bigger problem. This protects insurers and helps keep healthcare costs down for everyone.
- Remote Patient Health Monitoring
The agents of artificial intelligence in healthcare use big data analysis to keep track of patient’s health and look for early signs of trouble. This is especially helpful for patients who have just had surgery because they can be watched closely from home. When any issues are spotted early, healthcare providers can take action quickly, helping keep patients safe and improving their recovery.
- Better Diagnostic Determination
AI agents can be extremely helpful in diagnosing many diseases by analyzing test findings, patient symptoms, and medical imagery.
- Less Expenditure
Hospitals may save money using AI to manage everyday tasks such as patient registration, invoicing, and appointments. By managing these procedures more effectively, hospitals may reduce the number of managerial staff they require, freeing up finances for treating patients or innovative medical devices.
- 24 Hours Available
AI agents don’t need breaks or time off, making them available 24/7. This means your company can offer continuous service, support, or monitoring without interruptions. The consistent presence of AI agents ensures that all your support channels are covered at all times, providing reliable help whenever needed.
Future trends for AI agents in healthcare
With several major developments planned to shape their development and application in the upcoming years, the future of AI agents in healthcare is promising. The following are a few notable changes:
- Drug Discovery
AI is changing drug discovery by helping to identify possible drug candidates that are customized for certain patient groups and predicting the response of these medications based on each patient’s specific characteristics. AI in this sector has the potential to speed up drug discovery, provide safer medications, tailor medications for specific patient populations, and lessen side effects.
- Disease Detection
Because of AI, the diagnosis of autoimmune illnesses and other medical disorders is becoming faster. DNA sequencing can identify patients who are at high risk. This can assist in identifying patients who require extra care, diagnosing diseases early, enabling individualised treatment regimens, and lowering the proportion of patients who require hospital readmissions. Early illness detection allows medical professionals to treat patients more effectively and achieve better results.
Conclusion
As Healthcare autonomous agents continue to grow, their role in healthcare is becoming even more important. In the future, it will likely handle even more complex medical tasks, making healthcare faster, safer, and more personalized than ever before.
If you want to bring AI into your healthcare sector, Synergetics can help you make it smooth and effective. They offer creative and extraordinary platforms like Avatar Management, LangCertify, Agent Management, and many more to help you handle everything from specific patient records to imaging analysis. This means that healthcare can be more efficient with the help of these Synergetics AI platforms. To learn more about their product, check out their product video. Connect with Synergetics for unexceptional AI healthcare platforms.