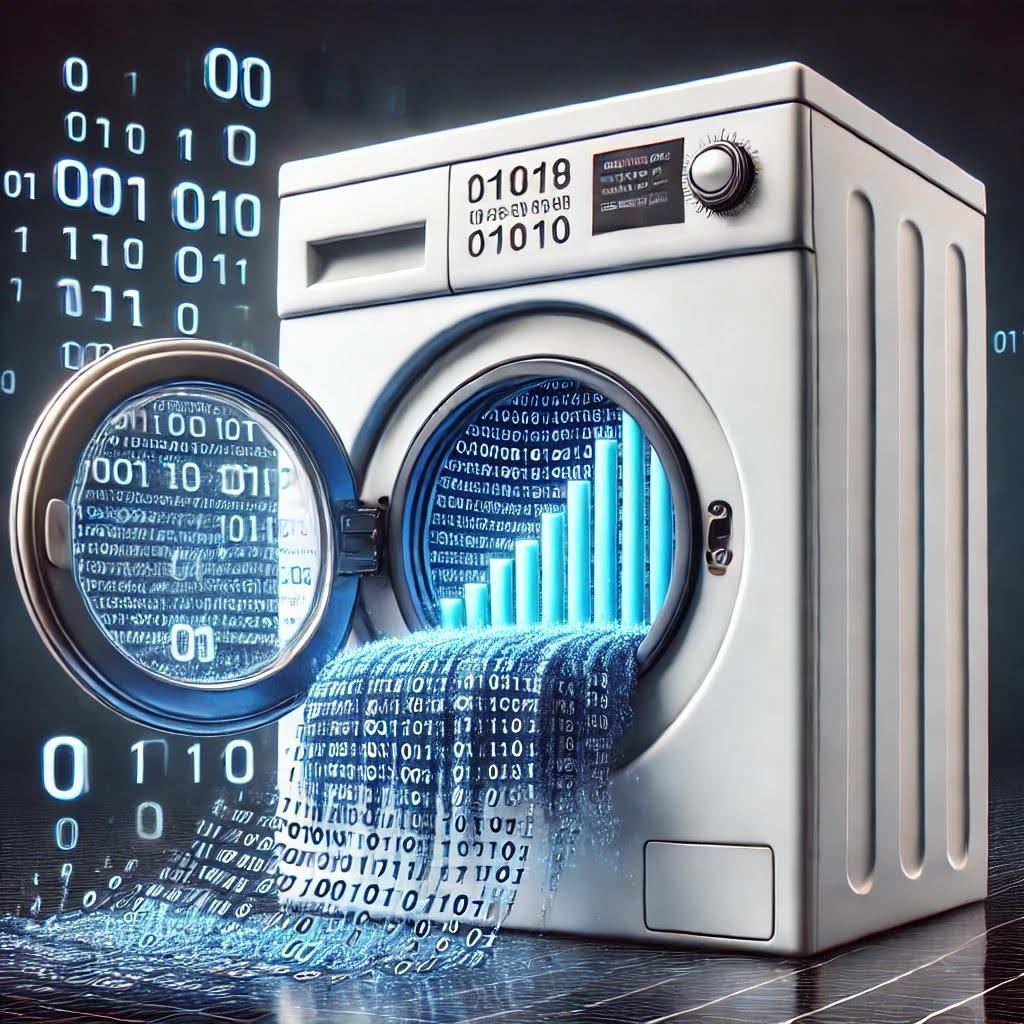
Welcome to another enlightening installment by the AI experts at Synergetics.ai, the leading agentic AI orchestration platform. In this article by Brian Charles, PhD, we dive deep into the critical role of data in artificial intelligence (AI) and explore contemporary practices that enhance AI solution development. Join us as we uncover the intricacies of collecting, cleaning, synthesizing, and managing data in the age of AI, and see how these practices are revolutionizing industries.
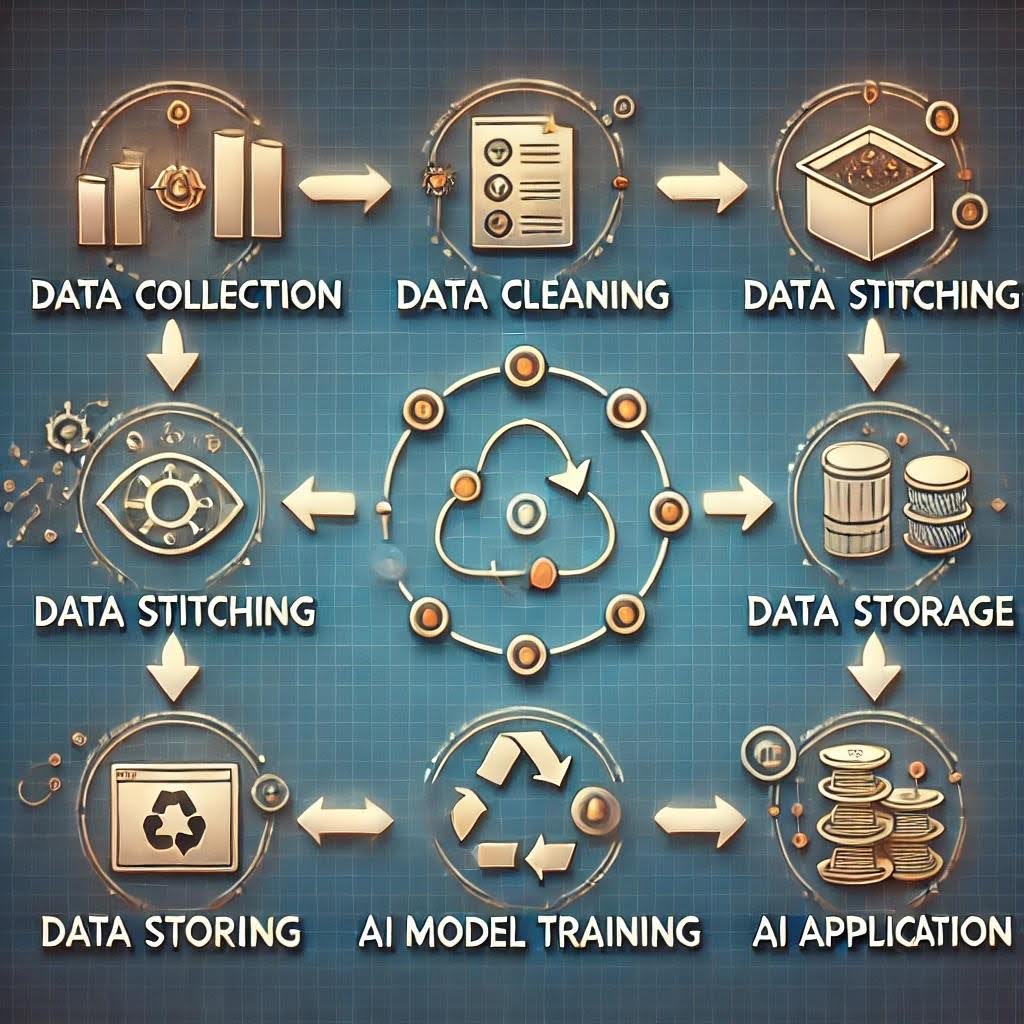
Collecting Data: The Bedrock of AI
Data collection is the foundation upon which all AI solutions are built. The quality and quantity of data significantly impact the effectiveness of AI models. Recent advancements in IoT (Internet of Things) have enabled the collection of vast amounts of real-time data from various sources. For example, smart cities are now leveraging IoT devices to gather data on traffic patterns, energy usage, and environmental conditions. This data is then used to optimize urban planning and improve the quality of life for residents.
However, collecting data is not without its challenges. Ensuring data privacy and security is paramount, especially with the increasing frequency of cyber-attacks. Companies must implement robust data governance frameworks to protect sensitive information and comply with regulations such as GDPR (General Data Protection Regulation).
Cleaning Data: Ensuring Quality and Accuracy
Raw data is often messy and requires cleaning before it can be used effectively. Data cleaning involves identifying and correcting errors, filling in missing values, and removing duplicates. This process is crucial for ensuring the accuracy and reliability of AI models.
In 2023, a major financial institution faced a significant data breach that resulted in corrupted data. Indeed, such occurrences are happening every day and may have already impacted your industry. In this case, the financial company’s AI-driven fraud detection system struggled to identify fraudulent activities due to the compromised data quality. This incident highlighted the importance of rigorous data cleaning processes. By implementing advanced data cleaning techniques, the institution was able to restore data integrity and enhance the performance of its AI systems.
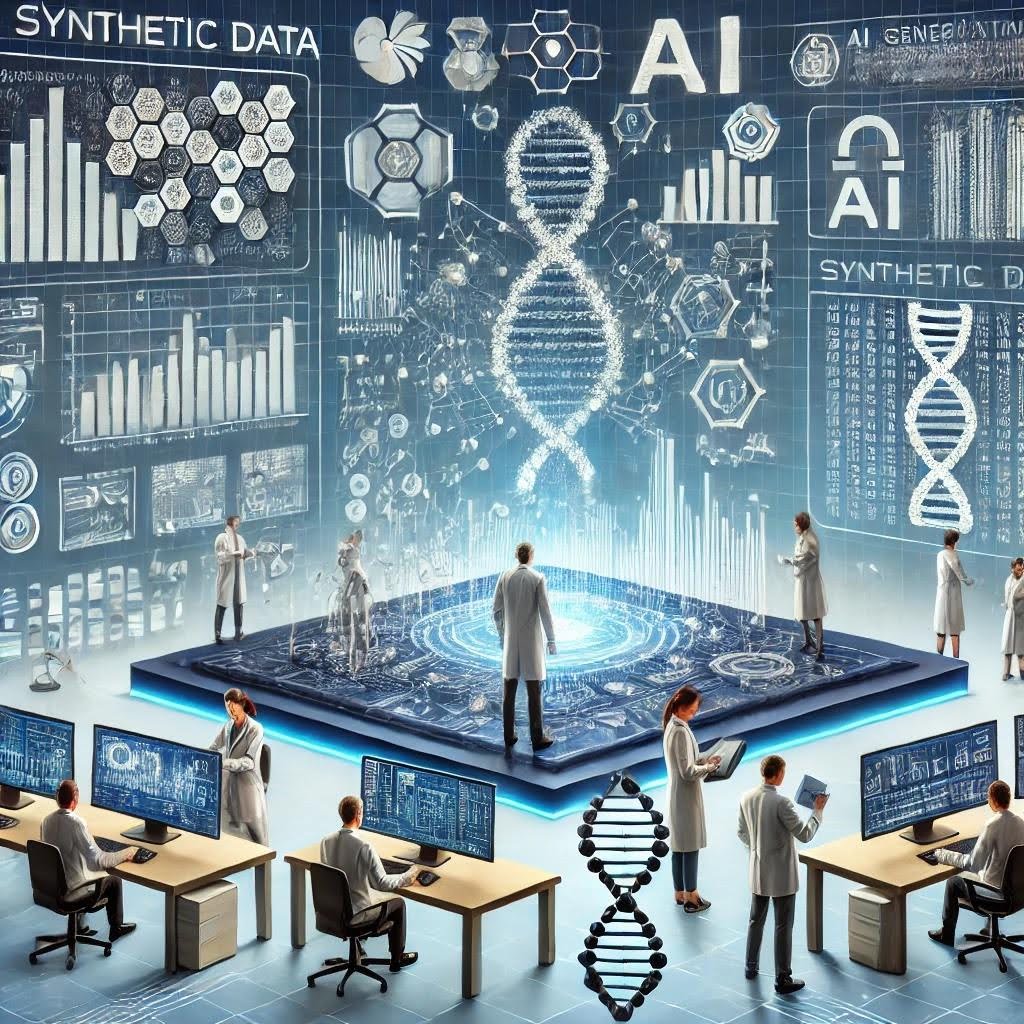
Making Synthetic Data: Bridging the Gaps
When real data is scarce, synthetic data can fill the void. Synthetic data is artificially generated and can be used to train AI models when real-world data is limited or unavailable. This approach is particularly useful in fields like healthcare, where patient data is sensitive and difficult to access. Recently, synthetic data has been used to simulate clinical trials, allowing researchers to test new treatments without compromising patient privacy.
One notable example is the use of synthetic data in autonomous vehicle development. Companies like Tesla and Waymo generate vast amounts of synthetic driving data to train their self-driving algorithms. This enables them to test scenarios that might be rare or dangerous in the real world, accelerating the development of safe and reliable autonomous vehicles.
Stitching Data: Creating a Unified View
Data stitching involves combining data from multiple sources to create a comprehensive and unified view. This practice is essential for gaining holistic insights and making informed decisions. For instance, in the retail industry, companies stitch data from online and offline channels to understand customer behavior better and optimize their marketing strategies.
Amazon, for example, uses data stitching to integrate purchase history, browsing behavior, and customer feedback. This unified data view allows Amazon to provide personalized recommendations and improve customer satisfaction. The result is a seamless shopping experience that drives customer loyalty and increases sales.
Storing Data: Scalability and Accessibility
Efficient data storage solutions are crucial for handling the massive volumes of data generated in the digital age. Cloud storage has emerged as a scalable and cost-effective solution, allowing organizations to store and access data from anywhere in the world.
Google Cloud’s BigQuery, as just one example, offers a serverless and highly scalable data warehouse designed for large-scale data analytics. This platform enables businesses to analyze petabytes of data quickly and efficiently, providing valuable insights that drive innovation and growth. Companies across various industries are leveraging cloud storage to streamline their operations and enhance their AI capabilities.
Embracing Contemporary Data Practices
The dynamic nature of data in the context of AI requires continuous adaptation and innovation. Contemporary practices such as real-time data processing, edge computing, and federated learning are pushing the boundaries of what AI can achieve. These advancements enable faster decision-making, reduce latency, and enhance data privacy.
For instance, edge computing allows data to be processed closer to its source, reducing the need for data to travel to centralized servers. This is particularly beneficial for applications requiring low latency, such as autonomous drones and real-time medical monitoring systems.
Conclusion: The Future of Data in AI and on Your Business
As we continue to advance in the field of AI, the importance of data cannot be overstated. Collecting, cleaning, synthesizing, stitching, and storing data are fundamental practices that enable AI to reach its full potential. By adopting these contemporary data practices, organizations can unlock new opportunities, drive innovation, and stay ahead in an increasingly competitive landscape.
At Synergetics.ai, we are committed to helping businesses harness the power of data to create intelligent, adaptive, and transformative AI solutions. Stay tuned for more insights and thought-provoking discussions as we explore the ever-evolving world of AI and its impact on various industries.